KEYNOTE SPEAKERS
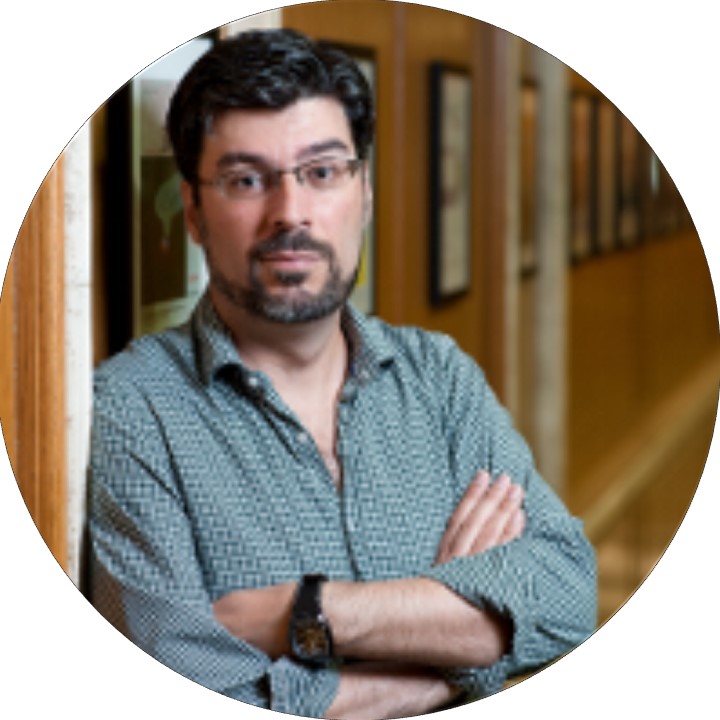
Luís A. NUNES AMARAL
Northwestern University, USA
An opinionated evaluation of where we are after a quarter century of complex networks
Luís A. N. Amaral is a Portuguese physicist recognized for his research in complex systems and complex networks. His specific research interests include the emergence, evolution, and stability of complex social and biological systems. He is best known for his work in network classification and cartographic methods for uncovering the organization of complex networks. He is currently professor at McCormick School of Engineering and Feinberg School of Medicine, Northwestern University.
Amaral received both his bachelor's and master's degrees in Physics from the University of Lisbon in 1990 and 1992, respectively. He then went to Boston where he performed his doctoral work under the supervision of H. Eugene Stanley at Boston University, and received his doctoral degree in 1996. He was a post-doctoral researcher at Forschungszentrum Jülich, MIT, Boston University, and Harvard Medical School. In 2002, he became a professor at Northwestern University.
Amaral has published over one hundred fifty scientific peer-reviewed papers in leading scientific journals, with more than twelve thousand citations. His research has been featured in many media sources. He is currently editor of several academic journals including Journal of Statistical Mechanics: Theory and Experiment and PLoS ONE. He also serves as the Graduate Program Director at the Department of Chemical Engineering at Northwestern University. He will become the co-director of Northwestern Institute on Complex Systems (NICO) in the fall of 2013.
-
It has been nearly 25 years since the seminal paper by Watts and Strogatz. In this period, the study of complex networks has fostered tremendous advances in our mathematical understanding of the structure, dynamics, and formation of complex networks. Those mathematical advances have made inroads into the work of scholars in biological, technological, and social studies. I speculate on how the community of researchers studying complex networks can have the greatest impact on the creation of new knowledge.
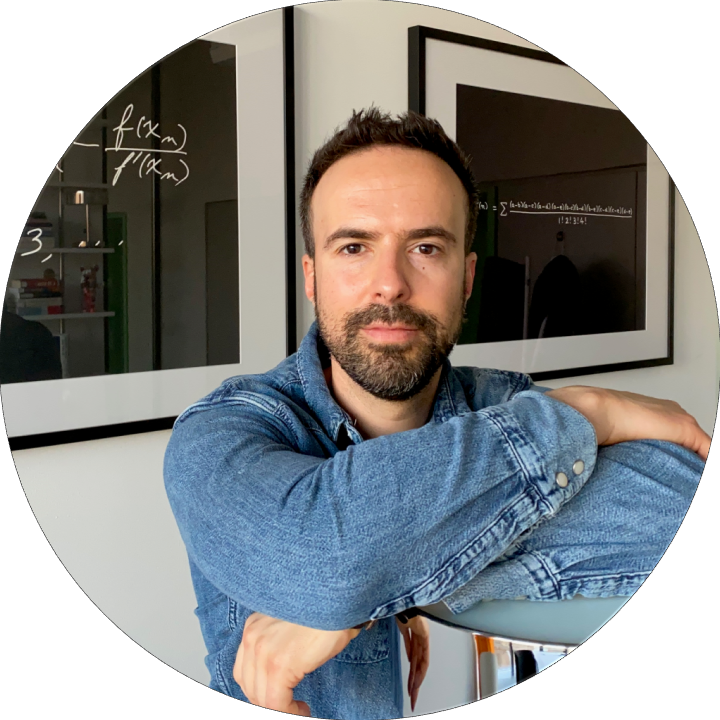
Manuel CEBRIAN
Max Planck Institute for Human Development, Germany
Networked and Crowdsourced Response to Time-critical Threats
Manuel Cebrian is a Max Planck Research Group Leader at the Max Planck Institute for Human Development, where he leads the Digital Mobilization Research Group. Previously, Manuel held positions at the Massachusetts Institute of Technology, CSIRO, and the University of California, San Diego. Manuel's research examines computational methods to create incentives that mobilize large groups of people to collaborate in solving major social challenges under time pressure. His current focus is on networked and crowdsourced responses to time-critical threats such as biological weapon attacks, terrorism, misinformation, radicalization campaigns, and cyberwarfare. His published papers appear in Science, Nature, the Proceedings of the National Academy of Sciences of the USA, and other peer-reviewed journals and proceedings in computer science and computational social science.
-
This seminar explores network and crowds' physical, behavioral, and computational limits for solving time-critical problems. I describe several real-world episodes in which we used social networks to mobilize the masses to deter threats of unprecedented complexity. From finding red weather balloons hidden across the United States, to tracking down thieves in a global hunt, to reconstructing shredded classified documents, to disabling a harmful Artificial Intelligence, the potential of crowdsourcing is real, but so are the exploitation, sabotage, and polarization dynamics that undermine the power of crowds. Keeping our communities, organizations, and institutions safe depends on harnessing the networks and crowds' power and talent while defending themselves from their aggressors.
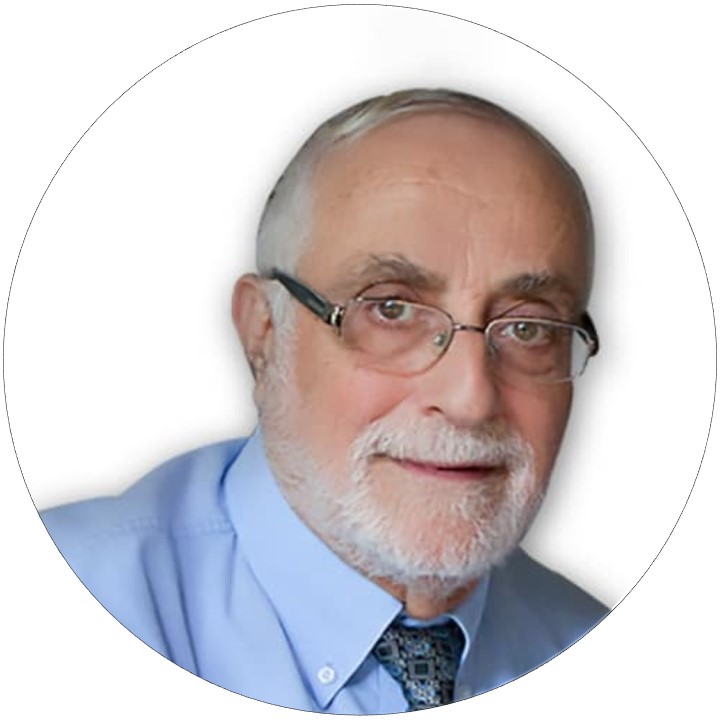
Shlomo HAVLIN
Bar-Ilan University, Israel
This keynote is sponsored by Applied Network Science, Springer
Network Science and Applications
Prof. Shlomo Havlin has made fundamental contributions to the physics of complex systems and statistical physics. These discoveries have impacted many other fields such as medicine, biology, geophysics, and more. He has over 60,000 citations on ISI Web of Science and over 100,000 in Google Scholar. His h-index is 111 (140) in Web of Science (Google Scholar). Havlin has been a Highly Cited Scientist in the last 3 years.
He is a professor in the Physics Department at Bar-Ilan University. He received his Ph.D. in 1972 from Bar Ilan University and he has been a professor at BIU since 1984. Also, between the years of 1999 – 2001 he was the Dean of the Faculty of Exact Sciences and from 1996 to 1999 he was the President of the Israel Physical Society.
Havlin is an IOP Honorary Fellow (2021), he won the Israel prize in Physics (2018), Order of the Star of Italy, President of Italy (2017), the Rothschild Prize for Physical and Chemical Sciences, Israel (2014), the Lilienfeld Prize for “a most outstanding contribution to physics”, APS, USA (2010), the Humboldt Senior Award, Germany (2006), the Distinguished Scientist Award, Chinese Academy of Sciences (2017), the Weizmann Prize for Exact Sciences, Israel (2009), the Nicholson Medal, American Physical Society, USA (2006) and many others.
His main research interests are in the field of statistical physics and complex networks, with a recent focus on interdependent networks, cascading failures, networks of networks and their implications to real world problems. The real-world systems he network application include physiology, climate, infrastructures, finance, traffic, earthquakes, and others.
-
Network science has been applied in many worldwide systems and processes in different disciplines. These include social systems, physiology, traffic, climate, epidemics, and very recently physics. I will show some examples of how network tools can mitigate pandemics [1], how switching between topics of scientists affects their scientific impact [2], and how fresh teams can be associated with original and interdisciplinary research [3]. I will also show how network tools can help to improve urban traffic [4], identify novel features in interdependent networks [5], and identify novel physical processes [6].
References:
[1] Cohen et al, PRL 85, 4626 (2000); PRL 86, 3682 (2001);
Y. Liu et al National Sciernce Review 8 (1) nwaa229 (2021)
[2] An Zeng et al, Nature Communications, 10, 3439 (2019)
[3] An Zeng et al, Nature Human Behavior, 5 (10), 1314-1322 (2021)
[4] Daqing Li et al, PNAS 112, 669 (2015); Limiao Zhang et al, PNAS 116, 8673 (2019);
G. Zeng et al, PNAS 116, 23 (2019)
[5] A Bashan et al, Nature Physics 9, 667 (2012); Y Berezin et al Scientific Reports 5 (1), 1-5 (2015)
[6] I Bonamassa et al, To be published (2022)
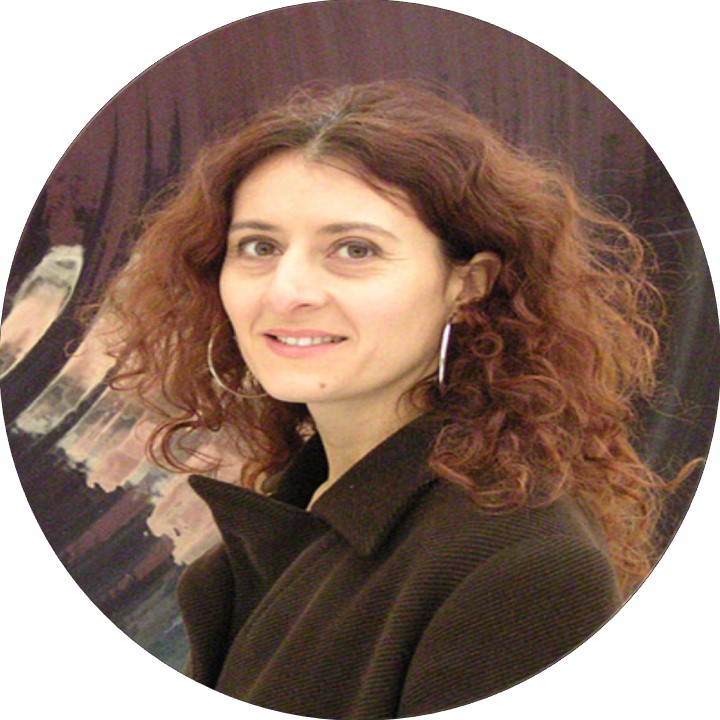
Information diffusion in trading networks
Giulia Iori is Professor of Economics at City University, London. Giulia has been a leading contributor to the development of an approach to complexity in financial markets and economic networks derived from the tools of statistical mechanics and has been a pioneer in applying Agent Based Models to Economics. Recent contributions include the analysis of the mechanisms that lead to large fluctuations in financial markets, the investigation of the role of the interbank market for the stability of the banking system, the analysis of high frequency financial time series, and some aspect of option pricing and hedging.
Her research has been funded by the European Commission, the EPSRC, and the British Academy. She was awarded the Lamfalussy Fellowship by the European Central Bank in 2003. She is the author of more than 50 publications on leading journals in Economics, Finance and Physics and regularly presents her work at international conferences as a keynote or invited speaker. She is the Co-Editor of Journal of Economics Dynamics and Control and Journal of Economic Interaction and Coordination and Associate Editor of Journal of Statistical Physics. She is a member of the London Mathematical Society and of the Institute of Physics.
-
In this talk I will presents results from recent work, with my co-authors, which explores experimentally how private information percolates through Over the Counter (OTC) markets. Despite the relevance of OTC markets, very few studies have explored how the distribution of trading links affetcs the way in which uninformed traders learn the private information of insiders, influencing in turn the efficiency and fairness properties of these markets. We constrain trading to happen on three predetermined network structures, namely the ring, the small-world, and the Erdos-Renyi random network. This allows us to introduce heterogeneity in nodes centrality and clustering, as well as to change the network average diameter, while keeping the density of the network fixed. In doing so we can isolate the effect of changes in the network structure on the trading behaviour of market participants, on traded prices, and on the distribution of traders’ profits.
We complement laboratory experiments with extensive computational experiments via a behavioural agent-based model (ABM). By calibrating the ABM model to the experimental data, we can specify the learning rule and shed light on roles of clustering and centrality on learning.
Our experiments provide support to the theory that information diffusion is affected by the network structure and by the number and position of insiders.
We show that learning is a collaborative, network process, enabled by synergistic interactions rather than by independent, pairwise interactions with better informed agents, and eased by clustering rather than by node degree or centrality. While regular, clustered, networks strengthen learning locally around the insiders, the higher closeness centrality of random networks better supports its diffusion. The competition between the speed of individual learning and the speed at which information spreads to others determines which market is more efficient and fair. We show that clustered networks can dominate random networks in some cases.
We find that information remains more localized, and is more accurate, around the insider in clustered networks, and more dispersed, but less precise, in random networks. As a result, markets with lower average misbelief may lead to more efficiency but not necessarily to more equality in profits.
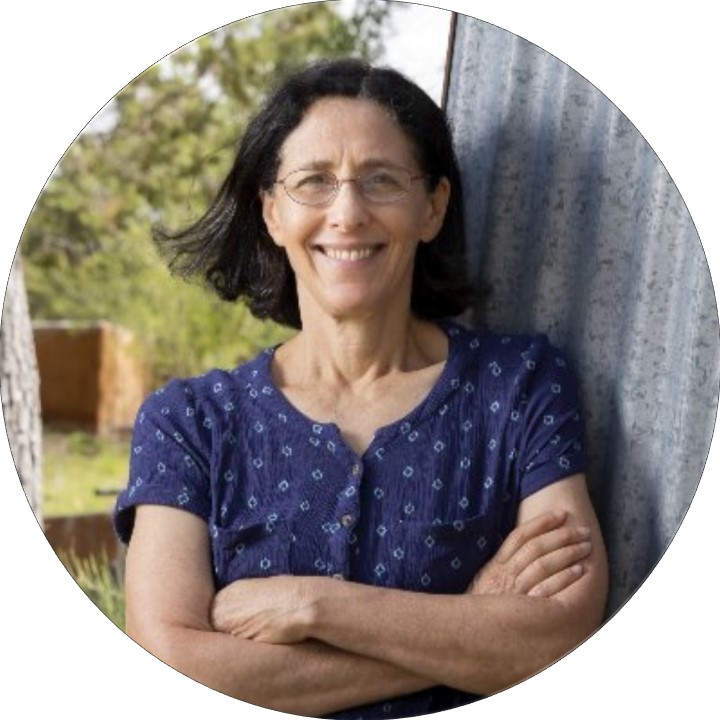
Melanie MITCHELL
Santa Fe Institute, USA
This keynote is sponsored by Applied Network Science, Springer
Why AI is Harder Than We Think
Melanie Mitchell is the Davis Professor at the Santa Fe Institute. Her current research focuses on conceptual abstraction, analogy-making, and visual recognition in artificial intelligence systems.
Melanie is the author or editor of six books and numerous scholarly papers in the fields of artificial intelligence, cognitive science, and complex systems. Her book Complexity: A Guided Tour (Oxford University Press) won the 2010 Phi Beta Kappa Science Book Award and was named by Amazon.com as one of the ten best science books of 2009. Her latest book is Artificial Intelligence: A Guide for Thinking Humans (Farrar, Straus, and Giroux).
Melanie originated the Santa Fe Institute's Complexity Explorer platform, which offers online courses and other educational resources related to the field of complex systems. Her online course “Introduction to Complexity” has been taken by over 25,000 students, and is one of Course Central’s “top fifty online courses of all time”.
-
Since its beginning in the 1950s, the field of artificial intelligence has cycled several times between periods of optimistic predictions and massive investment (“AI Spring”) and periods of disappointment, loss of confidence, and reduced funding (“AI Winter”). Even with today’s seemingly fast pace of AI breakthroughs, the development of long-promised technologies such as self-driving cars, housekeeping robots, and conversational companions has turned out to be much harder than many people expected.
One reason for these repeating cycles is our limited understanding of the nature and complexity of intelligence itself. In this talk I will discuss some fallacies in common assumptions made by AI researchers, which can lead to overconfident predictions about the field. I will also speculate on what kinds of new ideas and new science will be needed for the grand challenge of making AI systems more robust, general, and adaptable—in short, more intelligent.
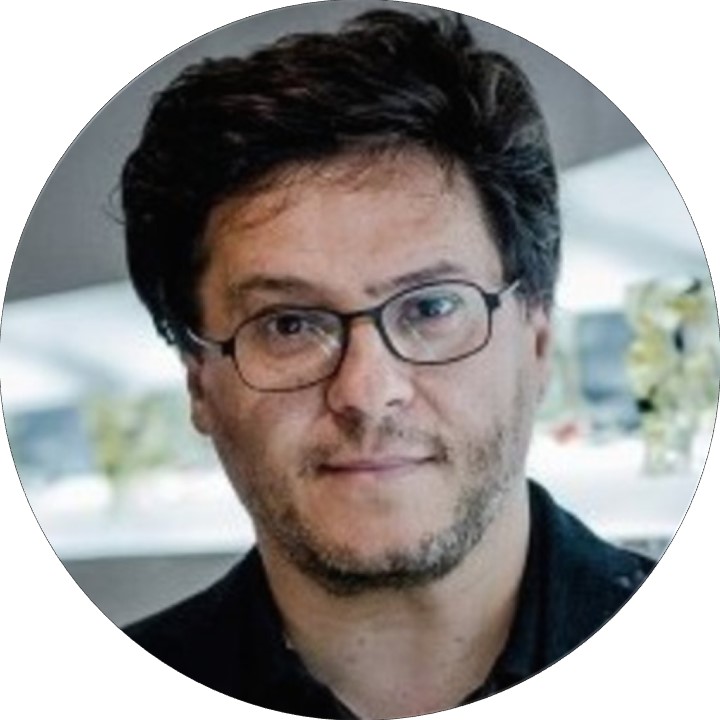
Evolutionary transitions: universality, complexity and predictability
I am ICREA research professor (the Catalan Institute for research and Advanced Studies) currently working at the Universitat Pompeu Fabra, where I'm the head of the Complex Systems Lab located at the PRBB. I teach undergraduate courses on Biomathematics, Biological Design and cComplex Diseases. I completed degrees in both Physics and Biology at the University of Barcelona and received my PhD in Physics at the Polytechnic University of Catalonia. I am also External Professor of the Santa Fe Institute (New Mexico, USA), Fellow of the European centre for Living Technology (Venice, Italy) and external faculty of the Vienna Complexity Hub. I have been awarded with grants from the James McDonnell Foundation and Advanced ERC grants.
-
The evolution of life in our biosphere has been marked by several major innovations. Such major complexity shifts include the origin of cells, genetic codes or multicellularity to the emergence of language, cognition or even consciousness. Understanding the nature and conditions for evolutionary innovation is a major challenge for evolutionary biology. Along with data analysis, phylogenetic studies and dedicated experimental work, theoretical and computational studies are an essential part of this exploration. With the rise of synthetic biology, evolutionary robotics, artificial life and advanced simulations, novel perspectives to these problems have led to an emerging new synthesis, where evolutionary innovations can be understood in terms of phase transitions, as defined in physics. Such mapping (if correct) would help in defining a general framework to establish a theory of evolutionary change and the role played by chance and constraints in shaping living complexity.